Awesome Python modules you probably aren’t using (but should be)
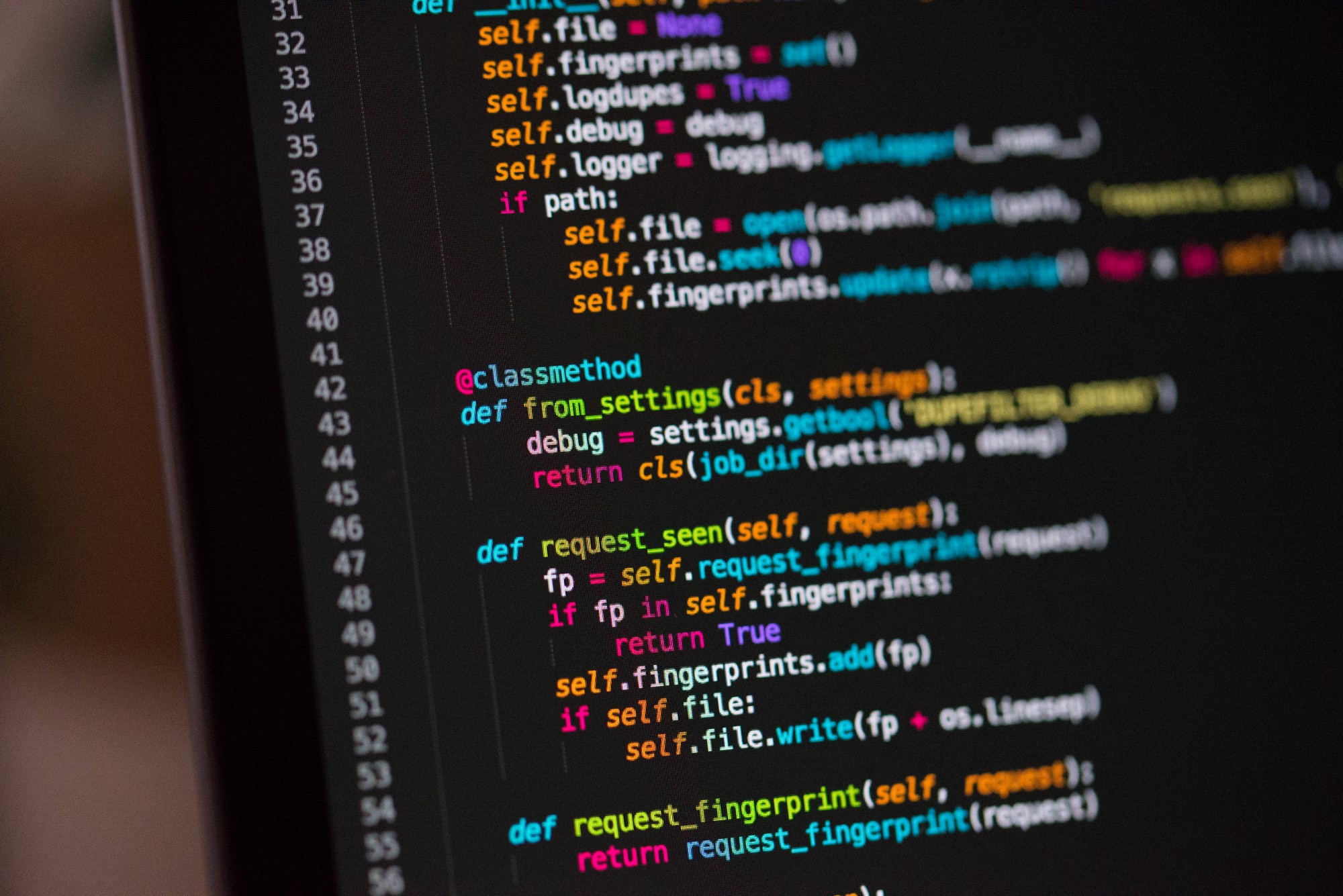
Python is a beautiful language, and it contains many built-in modules that aim to help us write better, prettier code.
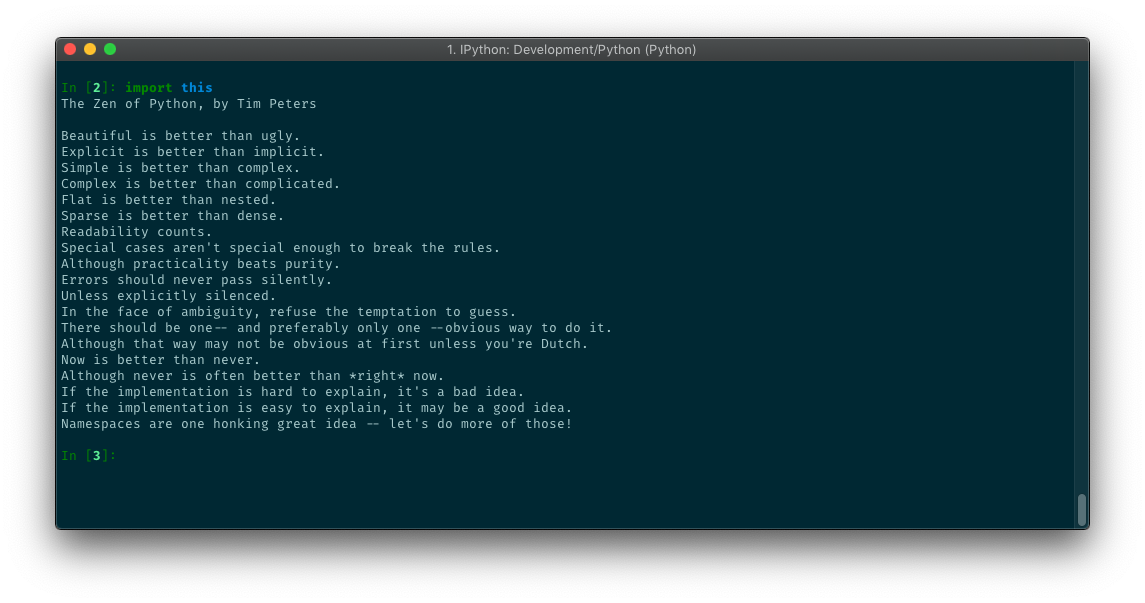
Objective
Throughout this article, we will use some lesser-known modules and methods that I think can improve the way we code - both in visibillity and in efficiency.
NamedTuple
I believe that some of you already know the more popular namedtuple
from the collections
module (if you don’t - check it out), but since Python 3.6, a new class was added to the typing
module: NamedTuple
.
NamedTuple
is actually a typed version of NamedTuple
, and in my opinion, it is more readable:
In [2]: import typing
In [3]: class BetterLookingArticle(typing.NamedTuple):
...: title: str
...: id: int
...: description: str = "No description given."
...:
In [4]: BetterLookingArticle(title="Python is cool.", id=1)
BetterLookingArticle(title='Python is cool.', id=1, description='No description given.')
Here’s the namedtuple
version:
In [6]: import collections
In [7]: Article = collections.namedtuple("Article", ["title", "description", "id"])
In [8]: Article(title="Python is cool.", id=1, description="")
Article(title='Python is cool.', description='', id=1)
Both classes are pretty similar and can save you and your fellow developers a lot of time trying to understand your code.
array.array
Efficient arrays of numeric values. Arrays are sequence types and behave very much like lists, except that the type of objects stored in them is constrained. - Python docs
When using the array
module, we need to instantiate it with a typecode, which is the type all of its elements will use. Let’s compare time efficiency with a normal list, writing many integers to a file (using pickle
module for a regular list):
In [9]: import array
In [10]: import pickle
In [11]: double_array = array.array("i", range(10 ** 6))
...: start_time = time.time()
...: with open("array_temp.bin", "wb") as f:
...: double_array.tofile(f)
...: array_end_time = time.time() - start_time
In [12]: int_list = list(range(10 ** 6))
...: start_time = time.time()
...: with open("list_temp.bin", "wb") as f:
...: pickle.dump(int_list, f)
...: list_end_time = time.time() - start_time
In [13]: print(f"It took {array_end_time} for int_array to complete")
...: print(f"It took {list_end_time} for int_list to complete")
It took 0.006399869918823242 for int_array to complete
It took 0.03600811958312988 for int_list to complete
In [14]: 0.03600811958312988 / 0.006399869918823242
Out[14]: 5.62638304213389
5 times faster. That’s alot. Of course it also depends on the pickle
module, but still - the array is way more compact than the list. So if you are using simple numeric values, you should consider using the array
module.
itertools.combinations
itertools
is an impressive module. It has so many different time-saving methods, all of them are listed here. There’s even a GitHub repository containing More Itertools!
I got to use the combinations
method this week and I thought I’d share it. This method takes an iterable and an integer as arguments, and creates a generator consisting of all possible combinations of the iterable with a maximum length of the integer given, without duplication:
In [16]: import itertools
In [17]: list(itertools.combinations([1, 2, 3, 4], 2))
[(1, 2), (1, 3), (1, 4), (2, 3), (2, 4), (3, 4)]
dict.fromkeys
A quick and beatiful way of creating a dict with default values:
In [18]: dict.fromkeys(["key1", "key2", "key3"], "DEFAULT_VALUE")
{'key1': 'DEFAULT_VALUE', 'key2': 'DEFAULT_VALUE', 'key3': 'DEFAULT_VALUE'}
Last but not least - the dis
module
The
dis
module supports the analysis of CPython bytecode by disassembling it.
As you may or may not know, Python compiles source code to a set of instructions called “bytecode”. The dis
module helps us handle these instructions, and it’s a great debugging tool.
Here’s an example from the Fluent Python book:
In [22]: t = (1, 2, [3, 4])
In [23]: t[2] += [30, 40]
---------------------------------------------------------------------------
TypeError Traceback (most recent call last)
<ipython-input-25-af836a8d44a2> in <module>
----> 1 t[2] += [30, 40]
TypeError: 'tuple' object does not support item assignment
In [24]: t
Out[24]: (1, 2, [3, 4, 30, 40])
We got an error - but the operation still succeeded. How come? Well, if we look at the bytecode (I added comments for the important parts):
In [25]: dis.dis("t[a] += b")
1 0 LOAD_NAME 0 (t)
2 LOAD_NAME 1 (a)
4 DUP_TOP_TWO
6 BINARY_SUBSCR
8 LOAD_NAME 2 (b)
10 INPLACE_ADD --> (value in t[a]) += b --> succeeds because list is mutable
12 ROT_THREE
14 STORE_SUBSCR --> Assign t[a] = our list --> Fails, t[a] is immutable.
16 LOAD_CONST 0 (None)
18 RETURN_VALUE